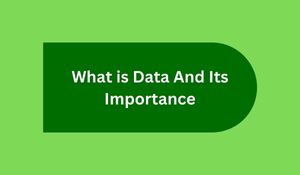
- Chapter : Overview – Data Science Tutorial for Beginners
- Chapter 1: What is data and the importance of data 2022?
- Chapter 2: Introduction to Data Science
- Chapter 3: Data Scientist vs Data Analyst vs Data Engineer: Job Role, Skills, and Salary
- Chapter 4: Top 15 Data Science Tools Everyone Should Know in 2022
- Chapter 5: Data Science with R: Getting Started
- Chapter 6: Getting Started With Linear Regression In R
- Chapter 7: A Guide to Time Series Forecasting in R You Should Know
- Chapter 8: How to Build a Career in Data Science
- Chapter 9: How to Become a Data Scientist in 2022
What is data and the importance of data 2022?
Since the introduction of computers, people have used the term data to refer to computer information. What is data? Data can be words printed on paper, bytes, and bits saved in electronic device memory.
What is Data in Data Science?
There is always data before anything else. Data is the cornerstone of data science; it serves as the foundation for all analysis. There are two forms of data in the context of data science: standard data and big data.
Standard data is structured data maintained in databases that analysts can control from a single computer; it is in table format and contains numeric or text values. We’re introducing the term “standard” for clarity’s sake. It emphasizes the difference between big data and other sorts of data.
Big data, on the other hand, is more extensive than standard data and not in a minor way. Moreover, big data is typically dispersed across a network of computers and ranges from variety (numbers, text, photos, music, mobile data, and so on) to velocity (retrieved and processed in real-time) to volume.
Standard Data in Data Science
Relational database management solutions are used to store traditional data.
To that end, every data is pre-processed before it is ready for processing. This is a necessary set of procedures that converts raw data into a more intelligible and valuable format for subsequent processing. Common procedures include:
- Gather raw data and save it on a server: It is raw data scientists cannot immediately evaluate. This information can originate from surveys or the more common automatic data gathering paradigm, such as cookies on a website.
- Label the data by class: This entails categorizing or marking data points with the appropriate data type—for instance, numerical or category.
- Data Purification/Scrubbing: Managing inconsistencies in data, such as misspelled categories and missing values.
- Data Balancing: If the data is unbalanced in such a way that the categories include an unequal number of observations and are therefore unrepresentative, using data balancing procedures, such as extracting an equal number of observations for each type and preparing it for processing, resolves the problem.
- Data Shuffling: Rearranging data points to avoid undesirable patterns and enhance prediction performance in the future. Data scientists use it when, for example, the first 150 observations in the data come from the first 150 people who visit a website; the data isn’t randomized, and patterns develop due to sampling.
Big Data in Data Science
There is some overlap between standard data management methodologies regarding big data and data science, but there are also many variances. To begin with, big data is stored on several servers and is much more complicated.
Pre-processing is much more critical when doing data science with big data since the data is more complicated. However, some procedures are conceptually comparable to typical data pre-processing, which is inherent in working with data.
- Gather the data
- Label the data by type. Since it is so diverse, big data is not classified by numerical or categorical, but by text, digital picture data, digital video data, digital audio data, etc.
- Data Cleansing: Managing inconsistencies in data, such as misspelled categories and missing values
- Data masking: When gathering data on a large scale, the goal is to keep any confidential information in the data private while allowing for analysis and insight extraction. The approach involves masking the accurate data with random and bogus data, allowing scientists to analyze without jeopardizing sensitive information. Naturally, scientists can accomplish this with regular data, but with big data, the information may be considerably more sensitive, masking a lot more urgency.
Where does the data originate from?
Traditional data sources include simple customer records and historical stock price information.
Big data, on the other hand, is all around us. A rising number of businesses and sectors use and create big data. Consider internet networks such as Facebook, Google, and LinkedIn, as well as financial trading data. Temperature measurement grids in diverse geographical regions and machine data from industrial equipment sensors are examples of big data.
Who Handles the Data?
Data professionals who work with raw data and pre-processing, developing, and managing databases, may go by several names. Although their titles sound identical, there are significant variances in the functions they do. Think about the following.
Data Architects and Data Engineers (also known as Big Data Architects and Big Data Engineers, respectively) play critical roles in the data science industry. The former builds the database from the ground up; they plan how data will be accessed, processed, and consumed. As a result, the data engineer takes the work of the data architects as a starting point and processes (pre-processing) the accessible data. Then, they ensure that the data is clean, organized, and ready for the analysts to take over.
On the other hand, the Database Administrator is in charge of the data flow into and out of the database. In fact, with Big Data, the entire process is automated; thus, a human administrator is unnecessary. The Database Administrator is concerned chiefly with conventional data.
However, actual data science can begin once the data has been processed and the databases have been cleaned and structured.
Data Types and Applications
The advancement of technology, particularly in smartphones, has resulted in text, video, and audio being classified as data and online and log activity records. Unfortunately, the majority of this material is unstructured.
The phrase Big Data is used in data definition to denote petabytes or more extensive data. Nowadays, web-based eCommerce is widely used, and business models based on Big Data have evolved into seeing data as an asset in and of itself. As a result, big Data has various advantages, such as lower costs, more efficiency, increased sales, etc.
The meaning of data extends beyond its use in computing applications. When it comes to what data science is, it is a body made up of facts. As a result, diverse definitions of data exist in finance, demography, health, and marketing, resulting in varied responses to the question, What is data?
How Do You Analyze Data?
There are ideally two methods for data analysis:
Data Analysis in Qualitative Research
Because quality information consists of words, depictions, photos, objects, and occasionally photographs, data analysis and research in personal information perform somewhat better than numerical information. Obtaining knowledge from such entangled data is a complex operation; hence, it is typically used for exploratory research and data analysis.
Although there are other approaches to discovering patterns in written data, a word-based technique is the most trusted and widely used global method for data exploration and analysis. In qualitative research, the majority of the data processing is done manually. In this case, the professionals usually scan the available material and look for repetitive or commonly used terms.
Data Analysis in Quantitative Research
The primary stage in data research and analysis is to do it for inspection to transform nominal information into something meaningful. The preparation of data involves the following phases.
- Data Validation
- Data Editing
- Data Coding
In quantitative statistical research, the application of descriptive analysis consistently produces better results. However, the analysis is seldom sufficient to demonstrate the reasoning behind the figures. Still, it is critical to consider the appropriate data collection and analysis method relevant to your review survey and the tale specialists need to convey.
Businesses that want to succeed in a hypercompetitive environment must be able to study complex research data, deduce significant bits of knowledge, and adapt to changing market demands.
How Industries are Using Data
Here are some of the applications of data in various industries:
Marketing: It is one of the most prevalent applications for big data. Any sector that sells a product or service may use big data to improve its marketing approach. In addition, because so many individuals maintain online profiles, such as social media accounts, they may be valuable sources of information for businesses trying to tailor their products to a particular consumer base.
Retail and Wholesale: In addition to marketing, retailers and wholesalers may use data in various ways. Big data may assist organizations in identifying current employment needs and forecasting future staffing needs based on buying behaviors, business development, local events, and seasonal patterns.
Media and Entertainment: Data is frequently used by online entertainment businesses to propose material to consumers based on their watching history and online actions. A video streaming service, for example, may account for an individual’s watching history by recording which kind of movies the user most frequently clicks on. Then, the site may propose videos that promote comparable content or videos that other users of a similar demographic watch often based on their watching history. As a result, the site may retain engagement and boost income by promoting material relevant to the viewer’s interests.
Banking and Security: Data may be used to improve internet security in the banking and finance business. They may utilize big data to forecast cyber crimes such as identity theft and card fraud by examining their particular clients’ transaction histories. Understanding their clients’ transaction history allows them to discover strange purchase habits that might suggest a security breach.
Precision Medicine and Healthcare: Using big data, the healthcare industry may be able to enhance the quality of patient treatment. Healthcare professionals use big data to track a patient’s medical history and assess risk factors associated with sickness, medical treatments, and prescription consumption. As a result, practitioners can provide tailored therapy to their patients by collecting, maintaining, and evaluating a patient’s medical history.
Top Data Trends For 2022
In today’s market, data drives any firm in various ways. The essential developments in today’s expanding industry are data science, big data analytics, and artificial intelligence.
The data analytics sector is expanding rapidly as more firms use data-driven models to simplify their business operations. Organizations increasingly use data analytics to fuel fact-based decision-making, embrace data-driven models, and grow data-focused product offerings.
These evolving data trends might assist firms in dealing with numerous changes and uncertainties. So, let’s look at a few data trends ingrained in the business.
Trend-1: Scalable Artificial Intelligence
COVID-19 has altered the economic scene in several ways, and old data is no longer valid. So, instead of classic AI approaches, certain scalable and better Artificial Intelligence and Machine Learning techniques that can deal with small data sets are now available on the market.
These solutions are more adaptable, safeguard privacy, are significantly quicker, and deliver a faster return on investment. In addition, the combination of AI and Big Data has the potential to automate and minimize the majority of manual processes.
Trend-2: Agile Data and Analytics.
Digital innovation, differentiation, and growth are possible with agile data and analytics models. The purpose of edge and composable data analytics is to create a user-friendly, adaptable, and seamless experience using various data analytics, AI, and ML technologies. This will allow executives to integrate business insights and actions and stimulate collaboration, productivity, agility, and the evolution of the organization’s analytics skills.
Trend-3: Cloud Computing and Hybrid Cloud Solutions
The increased usage of hybrid cloud services and cloud computation is one of the critical data trends for 2022. Public clouds are less expensive but do not provide robust security. On the other hand, private clouds are more costly but secure. As a result, a hybrid cloud combines a public and private cloud, with cost and security matched to provide more adaptability.
Businesses can accomplish this through the use of AI and ML. In addition, hybrid clouds are transforming enterprises by providing a centralized database, data security, scalability, and much more at a lower cost.
Trend-4: Data Visualization
Data visualization has quickly seized the industry with growing market trends and corporate information. Data visualization is said to as the final mile of the analytics process, and it supports organizations in comprehending large amounts of complex data.
By adopting graphically engaging methods, data visualization has made it simpler for businesses to make choices. It impacts analyst technique by allowing data to be viewed and displayed in the form of patterns, charts, graphs, and so on. Because the human brain understands and retains pictures more, it is an excellent tool to forecast future trends for the company.
Trend-5: Ethics
The moment has come to act on data ethics. Responsible firms will proactively integrate their data and artificial intelligence activities with human values. This becomes a prerequisite for ethical data usage and risk mitigation. History has shown that wise, ethical data usage and no-harm regulations lead to more innovation.
Because of the fast pace of AI deployment and the convergence of global challenges, there is no longer a one-size-fits-all strategy for ethical data and AI usage. Instead, organizations have a chance to specify how they will create and ethically use data and AI in this quickly changing digital environment.
Trust and transparency must drive innovation, growth, and customer connections now more than ever, decreasing the potential for technology to hurt people—including biased facial recognition and discriminatory lending. We will see more significant business and government commitment and responsibility for ethical, responsible data and AI usage as we lead with ethics and integrity. Learn how to design a system with purposeful human touchpoints and why including ethical data and AI rules into your organization’s governance decreases risk.
Learn More About Data and Data Science
We know we sparked your curiosity, don’t we? After reading this introduction to data science lesson, you’re probably anxious to learn more. Examine all of our Data Science and Business Analytics courses to choose the one(s) that are ideal for you, and you’ll be mastering data science in no time.
Drop An Enquiry
Search
COURSE CATEGORIES
- Agile
- APIGEE
- Articles
- BIG Data&Hadoop
- Cloud Computing
- Cyber Security
- Data Science Tutorial
- DevOps
- Digital Marketing Tutorial
- ElasticSearch
- Fusion
- Fusion Middleware
- Hyperion
- IBM
- Interview Questions
- Java
- JIRA
- LANGUAGES
- Microsoft
- Mobile Applications
- Net Suite
- Networking
- Oracle
- PEOPLESOFT
- RPA
- RSA ARCHER
- SAP
- SAS
- SEO
- SIEBEL
- Testing
- Tibco
- Tutorials
- Uncategorized
- Web Center
- Web Methods
- Web Technologies
- Workday